Ensuring AI systems are built and used fairly can be challenging but is hugely important if the potential benefits of AI are to be realised.
Recognising this, the government’s white paper “A pro-innovation approach to AI regulation” proposes fairness as one of five cross-cutting principles for AI regulation. Fairness encompasses a wide range of issues, including avoiding bias that can lead to discrimination.
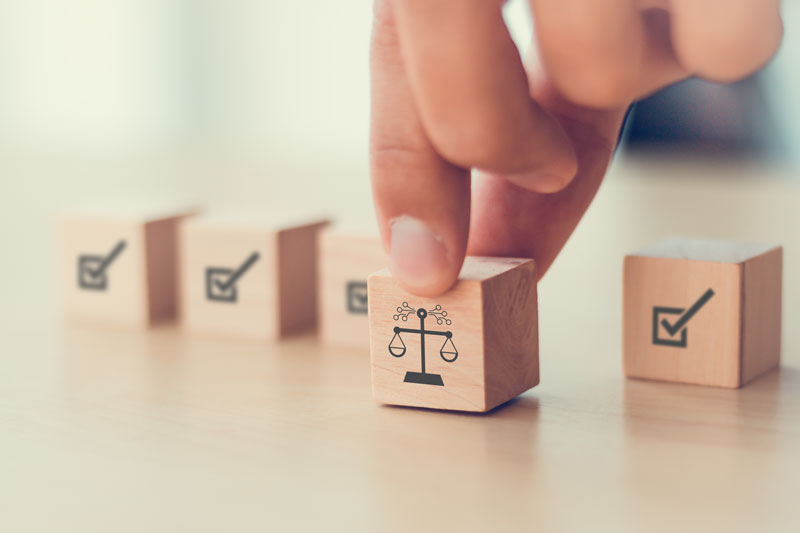
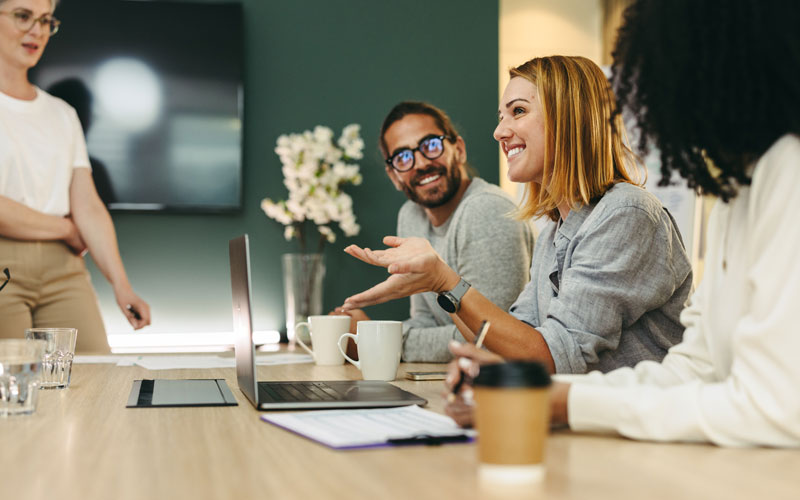
This issue has been a core focus for CDEI since we were established in 2018. Our 2020 “Review into bias in algorithmic decision making” provided recommendations for government, regulators, and industry to tackle the risks of algorithmic bias. In 2021, we also published the “Roadmap to an Effective AI Assurance Ecosystem”, which set out how assurance techniques such as bias audit can help to measure, evaluate and communicate the fairness of AI systems. Most recently, we published our report “Enabling responsible access to demographic data to make AI systems fairer”, which explored novel solutions to help organisations to access the data they need to assess their AI systems for bias.
Over this period, bias and discrimination in AI systems has been a strong focus across industry and academia, with significant numbers of academic papers and developer toolkits emerging. However, organisations seeking to address bias and discrimination in AI systems in practice continue to face a range of challenges, including:
- Accessing the demographic data they need to identify and mitigate unfair bias and discrimination in their systems.
- Determining what fair outcomes look like for any given AI system and how these can be achieved in practice through the selection and use of appropriate metrics, assurance tools and techniques, and socio-technical interventions.
- Ensuring strategies to address bias and discrimination in AI systems comply with relevant regulatory frameworks, including equality and human rights law, data protection law, and sector-specific legislation.
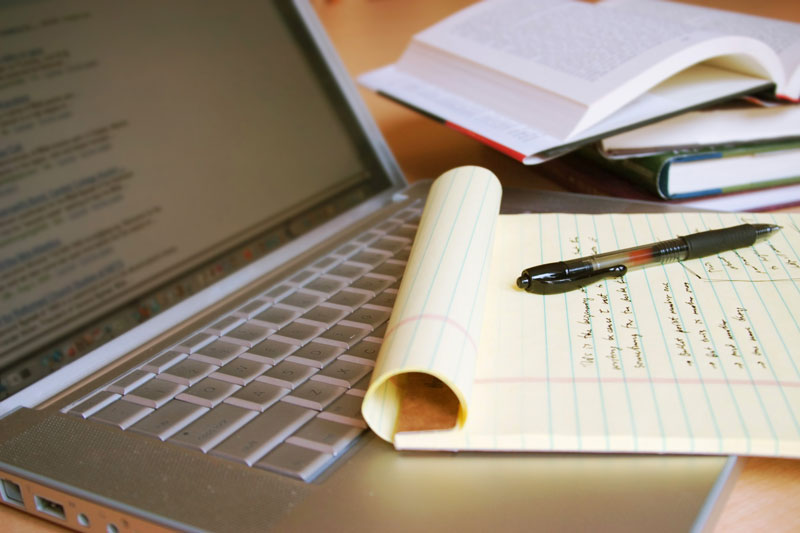
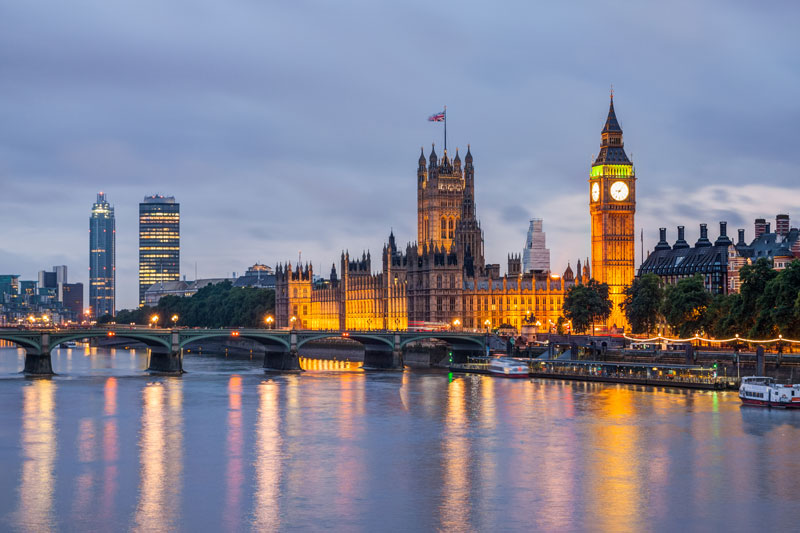
The Challenge will seek to address these issues by supporting the development of novel solutions to address bias and discrimination in AI systems. Winning solutions will implement socio-technical approaches to fairness, provide greater clarity about how different assurance techniques can be applied in practice, and test how strategies to address bias and discrimination in AI systems interact and can comply with relevant regulation.